Data Quality: How to Prepare Your Data for AI Implementation
83 percent of industry leaders now acknowledge that data quality is crucial for the success of AI-driven initiatives.
Yet, the reality on the ground is starkly different: more than half of businesses face significant revenue impacts due to data quality issues, with a notable increase in the average revenue affected. This year, the challenges have intensified, as the time to resolve data incidents has grown by 166%, and business stakeholders often find themselves identifying these issues first.
This underscores an urgent need for companies to not only adopt Artificial Intelligence (AI) to enhance operational efficiency but also to ensure that their data management strategies are robust enough to support successful AI implementation. Our blog will delve into why enhancing your data quality is more critical than ever and how you can prepare your data to make the most of AI technologies.
What is Data Quality
Data quality measures how well a dataset meets criteria for accuracy, completeness, validity, consistency, uniqueness, timeliness and integrity of data, and it is critical to all data governance initiatives within an organization.
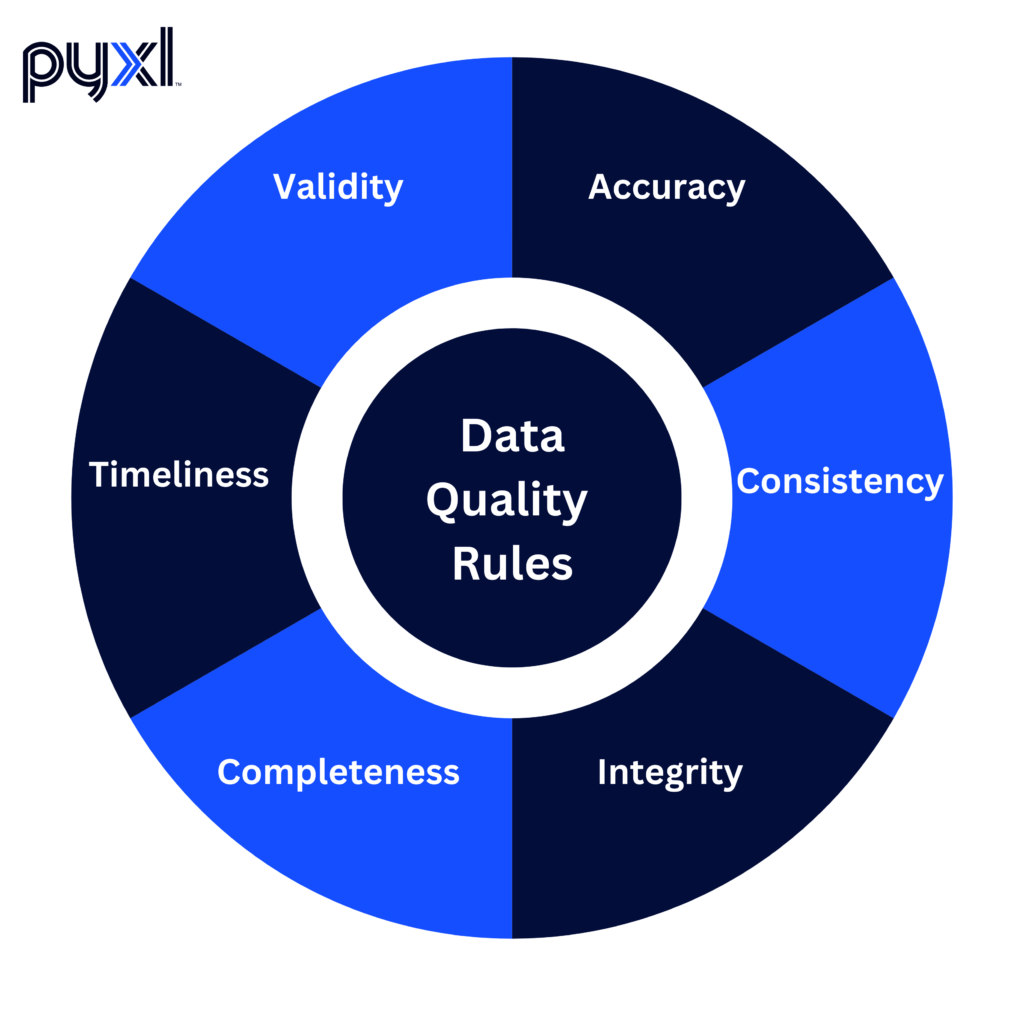
The Importance of Data Quality in AI
Since AI systems rely heavily on the data fed into them; the quality of this data directly influences their effectiveness and accuracy. Good data quality ensures that AI models perform as expected, making accurate predictions and providing reliable insights that businesses can trust for decision-making.
However, common data quality issues such as incomplete datasets, duplicate records, inaccuracies, and outdated information can severely impact AI outcomes. For example:
- AI models trained on incomplete data may develop biases or fail to capture the full scope of variables necessary for accurate predictions.
- Duplicate data can skew analysis results, leading to inefficient or erroneous decisions.
- Outdated information can result in recommendations that are no longer relevant, potentially costing businesses valuable time and resources.
- Inconsistencies in data, where different systems store similar data in conflicting formats, can further complicate data integration and analysis, making it challenging for AI systems to provide cohesive insights.
Addressing these issues is crucial to prevent “garbage in, garbage out” scenarios, where poor input data leads to unreliable outputs. Therefore, maintaining high standards of data quality is not merely beneficial but essential for leveraging AI effectively across business operations.
Data Preparation for AI
Effective AI implementation hinges on meticulous data preparation. Ensuring that your data is thoroughly collected, cleansed, and curated, lays the groundwork for leveraging advanced AI capabilities that drive insightful decisions and strategic actions.
Data Collection and Preprocessing
The foundation of any AI project is robust data collection. Gathering data from diverse sources enriches the dataset, providing a broader base from which AI can learn and make inferences.
Preprocessing is a critical step where this raw data is refined. Profiling the data helps identify anomalies or missing values that could skew results. For instance, missing data in customer purchase histories might lead to incorrect assumptions about buying preferences. Preprocessing includes tasks like normalizing data (scaling data within a specific range), handling missing values through imputation, and identifying outliers that might represent data entry errors or genuine anomalies.
Data Cleansing and Classification
Data cleansing ensures the reliability of your data. This step involves correcting inaccuracies and removing duplicates, which is crucial for maintaining the integrity of your AI models. Clean data leads to better, more accurate analytics.
Data classification is about understanding the sensitivity and relevance of your data. In a corporate environment, data might be categorized into public datasets that can be shared broadly and confidential datasets that require stringent access controls. Classification helps in applying the appropriate security measures and in compliance with data protection regulations.
Data Transformation and Validation
Transforming data for AI readiness might involve aggregating sales data to a suitable granularity or developing new features based on existing data, like calculating the lifetime value of customers based on their purchase history. This step is vital for preparing the data in formats that AI models can efficiently use.
Validation follows transformation to ensure the data maintains consistency and quality. This often involves checking for data integrity and consistency across different data stores to ensure that the transformation rules have been applied correctly.
Metadata, External Data Sources, and Storage Solutions
Metadata plays a crucial role in AI data management by providing information about the data’s origin, purpose, and structure, which is essential for effective data handling and usage.
Integrating external data sources—like market trends, demographic data, or economic indicators—can significantly enhance the predictive power of AI systems. The shift towards cloud-based storage solutions supports scalable, flexible, and cost-effective data management, crucial for handling large volumes of data generated today.
Data ethics and compliance are paramount, especially when handling sensitive information. It’s essential to ensure that data usage complies with legal standards like GDPR to protect consumer privacy and build trust.
Understanding and Curating Your Datasets
Being intentional in dataset selection is vital. Recognizing and utilizing common dataset types—such as categorical or numerical data—and understanding their characteristics helps in designing more effective AI models. Detecting and addressing gaps in datasets is crucial for thorough analysis and avoiding biased decisions based on incomplete data.
This comprehensive approach to preparing your data—from collection and cleansing to transformation and beyond—ensures your AI systems are built on a foundation of quality and integrity. This not only enhances their performance but also ensures they deliver reliable and actionable insights.
Unlocking AI’s Potential with Quality Data
Now that your data is clean and well-organized, your organization is perfectly positioned to unlock the transformative power of AI. High-quality data not only ensures the efficiency of AI operations but also opens up a myriad of possibilities for leveraging artificial intelligence across your business processes.
With this foundation, you can implement AI to enhance various functions such as predictive analytics, automated customer service, and intelligent automation processes. These AI-driven solutions can dramatically improve decision-making, streamline operations, and personalize customer interactions, ultimately leading to increased profitability and customer satisfaction.
For example, we partnered with Relat, to build an intelligent & personalized AI sales outreach assistant designed to allow business development and sales team members to deliver deeper and more meaningful outreach communications to prospects, customers and partners. Check out the full case study.
Key Takeaways
The journey from meticulous data preparation to AI implementation is pivotal for any organization aiming to harness the full potential of technology to enhance business operations. Quality data is not just a prerequisite but a powerful lever for activating advanced AI capabilities that can transform your business landscape—from streamlining operations to enhancing customer interactions and boosting sales performance.
Our custom-built AI solutions are designed to integrate seamlessly with your data, unlocking tailored benefits that elevate your company’s efficiency and competitiveness. Whether it’s optimizing lead management, enhancing personalization, or predicting market trends, our AI tools are crafted to meet your specific business needs.
Ready to explore how our custom AI solutions can transform your business? Visit our website to learn more and discover how you can start your journey towards AI-driven excellence today.
Updated: Nov 15, 2024